Supercharging Defense: AI Moves Beyond Threat Detection
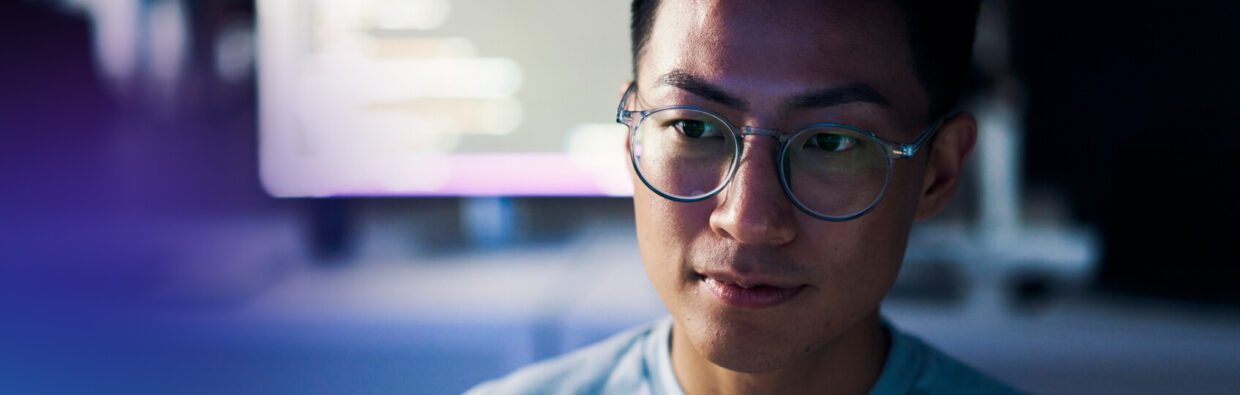
While currently the most visible, generative AI is far from the only exciting development in AI for cybersecurity. In the first blog in this series, we considered the evolution of generative AI and the state of AI in cybersecurity. For more than a decade, machine learning has been successfully used for threat detection: anomaly detection, malware detection, phishing detection, intrusion detection, endpoint detection. Today, most detection systems are powered at least partially by machine learning.
AI Capabilities that Assist Defenders
Besides detection, there are other, equally valuable use cases of machine learning appearing across the different SecOps disciplines. Perhaps the most powerful common thread of these applications is that they focus on localizing the power of threat intelligence, vulnerability data, and indeed other AIs to specific organizations. Using AI to model and predict attacker behavior is indeed critical—but understanding threats is only half the SecOps battle. Security teams need to be able to understand the environment to be protected, and emerging AI use cases help do just that.
Asset Criticality
Maintaining an up-to-date list of critical assets is a task that – as simple as it sounds – proves unrealistic for all companies. The IT landscape is increasingly complex and changes often. Machine learning can help identify critical assets based on how they are connected, how and by whom they are used and what processes they run. This enables an automated and continuous identification of critical assets localized to each organization.
Incident handling
Correct prioritization of an incident can decide whether a true positive alert is caught in time or stuck somewhere in a queue of benign positives. Using machine learning to predict – considering the specific environment – which incidents are more likely true positive than others can make the difference between these two scenarios.
Response automation
Machine learning can be used to identify response steps and actions for automation. Algorithms can identify common patterns of activities that experienced incident handlers perform during investigations. This helps improve accuracy and efficacy when selecting the parts of the response that can be automated.
Vulnerability management
In vulnerability management, machine learning can be applied for prioritization. Algorithms learn from past exploits and system behavior which vulnerabilities are most likely to be exploited so their patching is prioritized.
Much like for generative AI, these applications of machine learning support the human analysts to be more efficient and effective at their most time-consuming and most frequently executed tasks. One key point of these applications is that they can tailor SecOps to the operational and structural specifics of an organization.
A Double-edged Sword
Like so many technologies, AI has the potential to not only super-charge the defenders, but also the attackers. As cyber criminals increasingly use AI, we must be ready for an unprecedented level of sophistication and scale of targeted attacks.
Phishing and social engineering
Just like generative AI can be used to create training data for phishing detection, the same techniques can be used to produce highly targeted and realistic phishing campaigns. Beyond text-based phishing campaigns through email, generative AI can even be used to impersonate voice and video (also known as deepfakes) to a level that is hard to distinguish from the original. While not yet widespread, the first examples of successful social engineering attacks using deepfakes have already been reported.
Malware generation
With generative AI’s capability of generating code, the fear is that it enables less skilled cyber criminals to create new or modified malware. While we have seen successful proof-of-concepts of this, the extent to which this will become a reality is currently unclear.
Open source intelligence (OSINT)
Lastly, generative AI can be used to scan and analyze large amounts of publicly available data, extracting valuable information about potential targets. This can potentially save attackers significant time by gathering and summarizing information quickly, a process that would potentially take hours or days manually.
Conclusion
With the known and potential uses of AI by attackers, it is crucial to embrace and tap into the potential of the technology on the defender side – both what already exists today and what is rapidly being developed for tomorrow. Going well beyond detection, the truly valuable use cases of AI in SecOps are those that super-charge defense by combining the expertise and organizational knowledge of human defenders with the speed and depth of awareness of artificial intelligence, localizing the power of large-scale AI capabilities and tailoring it to the specific environment of an organization.